After the image generation feature of ChatGPT was released, a million users flocked within just one hour. As the function to generate portraits in the style of Japanese animation ‘Ghibli’ went viral, AI art spread across SNS and communities. However, behind the excitement lies the reality of GPU overload, power surges, and carbon emissions quietly accumulating. The invisible cost of creating a single image demands a balance between technology and the environment.
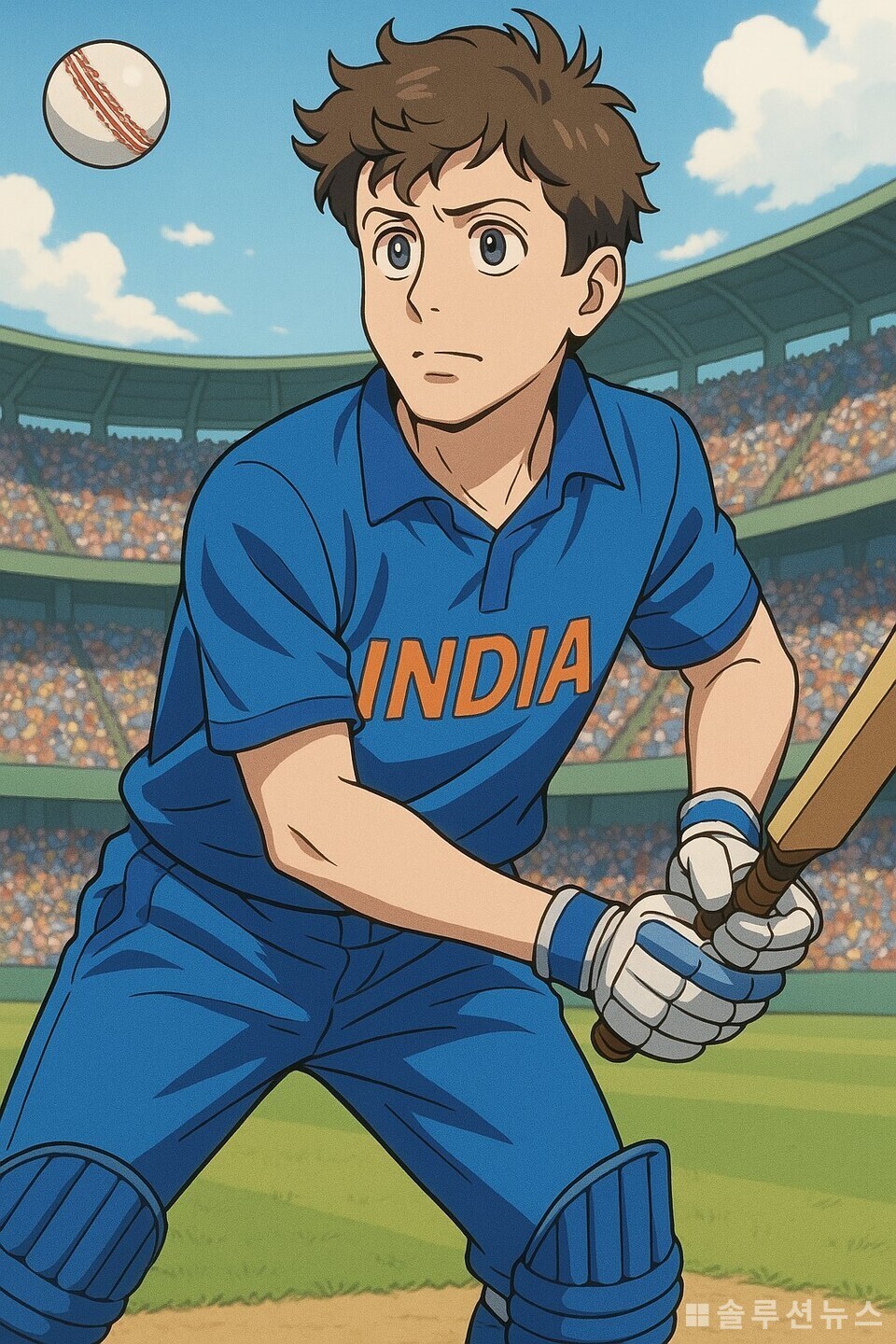
AI image generation is not just a simple calculation. To visualize the input text, massive parallel computations are required, and the device supporting this process is the ‘high-performance GPU’. As the usage of ChatGPT’s image function surged, some GPUs overheated and ceased operation, resulting in temporary service delays and outages. GPUs perform thousands of calculations per second, generating heat, and additional power is consumed to cool this heat.
Each time a prompt is executed, hundreds of matrix operations are repeated, with thousands of cores operating simultaneously. While users receive results in mere seconds, the physical systems experience significant load. The issue is not a one-time occurrence. AI models operate continuously year-round, and the same high load repeats with every new request.
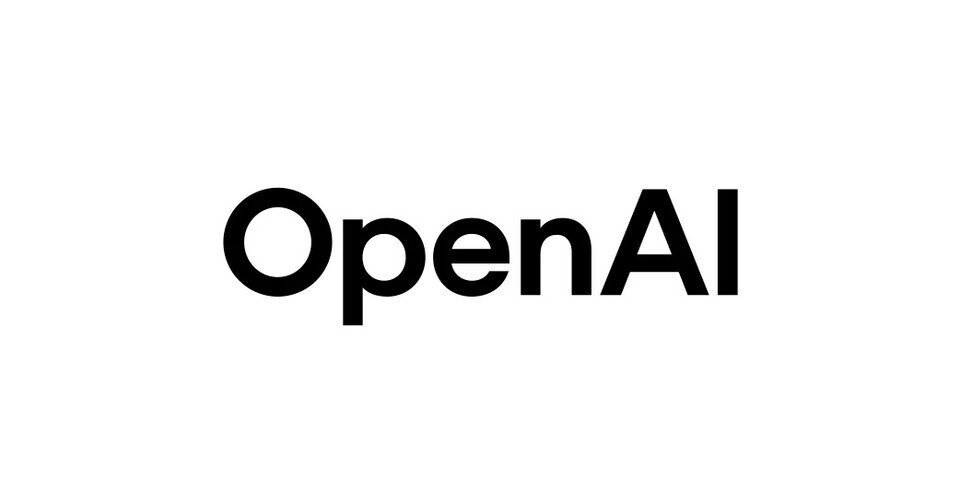
During the image generation process, users do not directly emit pollutants. However, every stage requiring computation, storage, and transmission is linked to physical power consumption. The power needed to train a single large AI model is known to match the energy usage of a typical household for one year. Systems running perpetually like ChatGPT’s image feature consume far more resources than one-time events.
According to some reports, large data centers consume over hundreds of thousands of kilowatt-hours per day. While OpenAI utilizes Microsoft’s cloud infrastructure, there are structural limitations to handling the rapidly escalating demand in the long term. The problem is that we are only in the early stages of AI adoption. Users continue to grow, and capabilities are becoming increasingly sophisticated.
Technical improvements such as more energy-efficient GPUs or optimization algorithms can be a meaningful short-term response. Some companies are declaring the development of ‘Green AI’, adopting lightweight models and energy-efficient infrastructures. However, as the computational demand itself rises, relying solely on technology to solve the problem has clear limits.
This is the time for policy intervention. Mandating energy ratings for data centers, introducing carbon taxes, and increasing renewable energy ratios can be means to distribute the external costs of AI technology socially. Some countries in the US and Europe have already introduced bills requiring the disclosure of energy usage by AI services. This is an issue that cannot be left solely to market autonomy; the establishment of norms and standards is required.
Users also need to adopt a responsible attitude. It’s necessary to reduce unnecessary image generation and refrain from repetitive experimental use. AI should be used efficiently, based on practical purposes and needs, rather than merely as an intriguing tool.
Technology has always pursued advancement. However, today’s AI must go beyond being faster and more sophisticated. Moving towards consuming less and assuming more responsibility is the true pathway for AI to become a tool for humanity. Depending on how we handle AI, technology can either open up the future or leave costs behind.